View the original RETHINK Retail article: How AI and Machine Learning are Revolutionizing Retail: Insights from Nextuple’s Co-Founder, Devadas Pattathil
In a recent interview with RETHINK Retail, Devadas “Das” Pattathil, Co-Founder and Head of Product at Nextuple, shared insights on how artificial intelligence (AI) and machine learning (ML) are reshaping the retail landscape.
The Role of AI and ML in Retail
“One area that is really emerging is predicting inventory availability, especially for retailers who use their stores for fulfillment.”
AI and ML have long been used in retail for fraud detection, but their application in inventory management and order fulfillment is still developing. According to Pattathil, “One area that is really emerging is predicting inventory availability, especially for retailers who use their stores for fulfillment.” This technology helps retailers overcome a common challenge: understanding how much stock is available to fulfill orders when inventory data from in-store and online systems can be inconsistent.
Pattathil explains that AI integrates signals—such as point-of-sale transactions and picker actions—to predict stock levels more accurately. This ensures shelves aren’t empty when customers visit the store, improving both customer experience and inventory turnover.
AI for Smarter Fulfillment
“Outcome-based sourcing is taking this one level higher where I don’t look at a single order. Rather, I look at a network.”
Pattathil also discussed “outcome-based sourcing,” where AI is used to manage an entire network of orders to optimize results, rather than focusing on individual orders. This approach balances factors such as fulfillment speed and cost. “Outcome-based sourcing is taking this one level higher where I don’t look at a single order. Rather, I look at a network,” Pattathil explained.
This allows retailers to make smarter fulfillment decisions, reducing waste by moving excess inventory to locations where it’s needed most.
Meeting Evolving Customer Expectations
“The omnichannel experience has exploded with the smartphone revolution.”
Today’s consumers expect a seamless shopping experience across multiple channels, whether in-store, online, or on their mobile devices. “The omnichannel experience… has exploded with the smartphone revolution,” Pattathil said. Shoppers now expect consistent prices, promotions, and inventory availability across all platforms, whether shopping online or in-store.
To meet these demands, Pattathil recommends that retailers establish centralized services that offer consistent pricing, promotions, and inventory across all channels. He also emphasized the importance of knowing omnichannel customers and personalizing their shopping experiences based on their complete purchase history.
Challenges in AI-Driven Order Management
“If you don’t have the right data, you’re not going to get the right outcomes.”
Transitioning to AI-driven order management comes with its challenges. Pattathil stressed the importance of clean data: “If you don’t have the right data, you’re not going to get the right outcomes.” Retailers must invest time to ensure they have adequate, clean data to train AI models effectively.
Explainability is another challenge. AI models need to be understandable by business stakeholders for successful adoption. “Many AI projects fail because business stakeholders don’t understand the outcomes,” Pattathil warned. He also highlighted the cost of AI implementation, recommending an iterative approach with proofs of concept (POCs) to validate the business case at each step.
AI’s Impact on Delivery Accuracy and Customer Satisfaction
“A precise promise boosts the customer confidence in the brand and obviously leads to better sales.”
AI plays a crucial role in improving delivery accuracy, which directly impacts customer satisfaction. AI can analyze transactional history to provide precise delivery estimates, rather than the conservative estimates retailers often use to avoid delays. “A precise promise… boosts the customer confidence in the brand and obviously leads to better sales,” Pattathil noted.
Even in cases of delays, AI can detect issues early and notify customers before the promised delivery date is missed, building trust and reducing friction.
Real-World Applications of AI in Retail
By leveraging AI, the retailer was able to predict delivery times with 99% accuracy.
Pattathil shared real-world examples of how AI-powered solutions have driven operational improvements. In one instance, a high-end retailer struggled with long vendor shipping times, which led to overly conservative delivery estimates. By leveraging AI, the retailer was able to predict delivery times with 99% accuracy, significantly improving customer satisfaction and sales.
Another example is “profitable promising,” where AI helps retailers balance the cost of fulfilling an order with the likelihood of conversion from a shopper’s cart to a completed purchase. This allows retailers to optimize both customer experience and profitability.
The Role of Generative AI in Order Management
Looking ahead, generative AI (GenAI) has the potential to transform customer service and supply chain management. Pattathil highlighted how GenAI could automate routine customer service tasks, such as answering questions about orders and returns, reducing costs while improving customer satisfaction. GenAI could also simplify the work of supply chain analysts, enabling them to query data more efficiently and gain actionable insights.
In closing, Pattathil emphasized the importance of explainability in AI-driven decisions, stating that it’s key to securing the support of business stakeholders and unlocking the full potential of AI in order management.
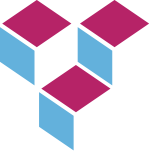