View the original Retail Customer Experience article: Best practices to transform retail order management with AI right now
To be successful in a highly competitive global retail environment, brands must anticipate customer needs, optimize order promising and inventory management and adapt quickly to consumer trends and preferences.
Today's shoppers are multifaceted. Like the American poet Walt Whitman, they are large and they contain multitudes.
In 2024, they don't just want to shop in-store, online, or on their phones. They want all of these options at different times. However, regardless of where they shop, they know what they want. They want the right item from the right channel at the right time.
This means that everything from in-store product availability to delivery times increasingly defines successful customer experiences.
It's a seemingly simple demand that retailers are struggling to deliver. According to one consumer survey, 78% of U.S. shoppers experienced out-of-stock items when shopping in-store over the last year, and 73% of online shoppers said they encountered this problem.
With brand loyalty in a free-fall, shoppers are quickly turning to competitors when brands can't reliably provide the inventory they crave.
Therefore, to be successful in a highly competitive global retail environment, brands must anticipate customer needs, optimize order promising and inventory management, and adapt quickly to consumer trends and preferences.
Artificial intelligence and machine learning capabilities will help brands achieve this with excellence and consistency.
Specifically, these technologies enable predictive order promising, conversion probabilities, and complex queries of inventory audit or reconciliation reports. Here's how.
Understanding the Models Impacting Retail
AI and ML are not singular technologies. They are multifaceted, big-tent technologies with many forms and iterations. Not all of them are helpful for brand building and inventory management.
However, several models can transform the way retail manages its inventory, allowing them to elevate promising and inventory management.
For starters, predictive order promising, which accurately predicts the processing lead times for various aspects of order fulfillment, including:
- Fulfillment centers
- Stores
- Drop-ship vendors
- Marketplace vendors
- Shipping transit times from carriers
By analyzing historical data to accurately predict how long it will take to deliver an item to a customer, predictive order promising can make real-time, instantaneous predictions for delivery dates.
Additionally, dynamic inventory management systems leverage expansive data sets, including historical demand patterns and sales goals, to produce adjustable inventory levels available to online and offline customers.
While traditional, static inventory systems rely on predetermined stocking levels revised through periodic updates, dynamic inventory optimization continuously monitors and adjusts inventory levels based on real-time demand signals and market fluctuations.
When coupled with Generative AI,co-pilots that allow business users to query data sets and decisions the system is making, inventory management teams have more insight into and control over their supply chains, enabling proactive adjustments and strategic decision-making.
Together, these AI and ML models allow companies to respond more dynamically to real-time market changes, creating better customer relationships and driving sales outcomes.
The Benefits of AI-powered Models
AI and ML models are helping companies convert their expansive data sets into actionable insights and proactive inventory adjustments that enhance holistic brand performance.
With the right predictive order promising model, retailers can expect conversion rate improvements from 5% to 20% and cost-to-serve reductions of 3% to 15%. With dynamic inventory models, they can reduce overall inventory by up to three percent and improve inventory-related cancels by up to 40%.
For example, one national wholesale club needed to update its enterprise inventory capabilities because lack of visibility caused issues with canceled orders and missed sales opportunities online. By updating its inventory tech stack to the latest solutions, the company moved inventory reservations into the checkout process, improved node controls with no picks, and eliminated static safety stock calculations.
In addition, the updated tech solution came with robust reporting, alerting, and reconciliation capabilities related to inventory data, which will shorten root cause analysis cycles.
More broadly, inventory managers can expect AI and ML models to deliver:
- Agility: Anticipate changes in consumer preferences and adjust inventory levels proactively
- Segmentation: Tailor inventory strategies to different market segments, addressing unique customer needs
- Cost efficiency: Avoid overstocking and stockouts
- Improved conversion through accurate and specific estimated delivery dates
- Risk reduction: Test predictive models on historical orders before deploying them, reducing risk and increasing confidence in decision-making
Of course, the actual benefits are as dependent on the technology implementation as on its capabilities, requiring leaders to thoughtfully and intentionally integrate this technology into their existing workflows and capabilities.
Consider this Before Updating to AI/ML Models
Modernizing complicated supply chain and order management systems with the latest AI and ML capabilities should be done carefully and intentionally.
Start by evaluating a solution's integration capabilities and understanding how well this new system will integrate with existing software and tools. Seamless integration can keep data flowing across all systems, while less-compatible solutions can create information silos that limit the impact of your inventory management strategies.
Similarly, account for the solution's scalability. Select an AI and ML solution that can grow alongside your brand.
Finally, ensure the data your models will be using is well understood by the organization. For example, if you are attempting to deploy an ML model to improve sourcing decisions, is the data set being used there fully baselined and understood by all teams?
To be sure, today's shoppers are complicated and choosy, with shifting preferences that can be difficult for retailers to keep up with. The best brands will tackle this challenge with technology ready to meet the moment.
In this way, the arrival of AI and ML solutions coincides perfectly with this challenge, allowing supply chain and inventory management teams to outpace competitors who can't or won't keep up with this changing landscape.
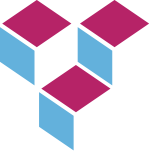