When it comes to omnichannel commerce, balancing customer expectations and optimizing inventory management is a constant challenge. One of the most important parts of this balancing act is the omnichannel Available-to-Promise (ATP)—the quantity of products available for sale at any given time online that considers the optimal level of safety stock at the store and for walk-in customers. Traditional methods for ATP calculations can fall short, especially in scenarios where demand forecasting is complex, or data is incomplete. That's where the power of machine learning (ML) steps in for better inventory management.
Why Getting ATP Right Matters
First, why is getting the ATP right such a big deal? Well, it boils down to a few key reasons:
- Happy Customers. Nobody likes showing up to a store or clicking "buy" online only to find out the item they want is out of stock. That's a recipe for frustration, lost sales, and even worse—a blow to your brand's reputation.
- Inventory Sweet Spot. Too much inventory is like money sitting on a shelf gathering dust (or worse, going bad!), while too little means missed sales opportunities. An ATP that is trying to optimize on fill rate performance at store—while maximizing offline and online sales—helps you find that perfect balance.
- Smooth Operations. When ATP is accurate, it ensures you have the right products in the right place at the right time.
Traditional ATP Calculations Can Be Improved
The traditional approach to ATP involves intricate calculations based on historical sales data, current inventory levels, and anticipated future demand. But several factors can make these calculations a bit messy:
- Inaccurate Forecasting. Historical sales data might not always reflect future demand due to factors like seasonality, promotions, or even unpredictable events.
- Data Gaps. Missing information on pricing, promotions, or out-of-stock events can significantly skew forecasts.
- Multiple Objectives. Businesses often have competing goals, like maximizing ATP to drive sales while simultaneously minimizing out-of-stock items to prevent customers from being unhappy.
The Challenge of Defining 'Optimal'
In our experience working with clients, we've found that defining "optimal ATP" isn't always straightforward. What may seem like a simple goal of maximizing ATP can quickly become complex when considering other key performance indicators like fill rate. Different teams within an organization may have varying priorities, leading to disagreements on the ideal metric to focus on.
A Data-Driven, Customer-Centric Approach
At Nextuple, we understand these challenges intimately. That's why we've developed a solution that empowers businesses to tailor their ATP strategy to their specific needs.
Our innovative approach harnesses the power of machine learning (ML) to address the shortcomings of traditional ATP methods. By analyzing vast amounts of data and identifying complex patterns, our ML-powered engine generates more accurate ATP calculations, even in the face of unpredictable events and data gaps.
We've also built a tool that allows businesses to weigh the importance of different factors, such as ATP and fill rate. This flexibility ensures that your ATP strategy aligns perfectly with your unique business objectives.
Additionally, our ML model can be used to forecast sales at an item/node level, providing a valuable comparison point against your existing forecasting models. This helps you gain a deeper understanding of your inventory dynamics and make more informed decisions.
The integration of machine learning into ATP calculations isn’t just a trend; it's the future of inventory management. By harnessing the power of data and advanced algorithms, businesses can unlock new levels of efficiency, customer satisfaction, and profitability.
Ready to optimize your ATP and revolutionize your inventory management? Contact Nextuple today to learn more about our ML-powered solution and schedule a demo.
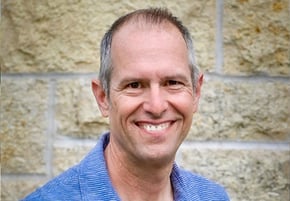